Testing Theories of Political Persuasion Using AI
In recent work, published in PNAS, we use AI to microtarget political messages.
Political Microtargeting
In the early 2010s, the British firm Cambridge Analytica came under fire for collecting personal information from millions of Facebook users. The public was horrified to learn that this information was used to target political and other ads. The idea that giant companies were stealing your data and using it to tailor persuasive messages to you was new and frightening. Nowadays, most of us are accustomed to the idea that the content we see is specifically for us. This isn't necessarily a bad thing. When you finish a show on a streaming service, getting a high-quality personalized recommendation is great. On the other hand, some are worried about the use of tailored content to manipulate the public at scale.
The Cambridge Analytica scandal happened before the advent of modern generative AI. With the accessibility of modern Large Language Models (LLMs) like ChatGPT, people can generate human-level text in real time. Given this technology, fears of population-scale real-time political microtargeting seem fairly reasonable.
Do we know, however, that political microtargeting is more persuasive than other forms of political advertising? The scientific literature on this topic is mixed, partially due to differing definitions of the phrase political microtargeting.
For some, political microtargeting is as simple as serving different ads to liberal and conservatives. Others might not consider it microtargeting unless the message is specifically tailored to several of the user's demographics like ethnicity, age, and religion.
I wanted to get to the bottom of this, so about a year ago I went to my co-authors and proposed this study. While multiple previous studies had tested single-message and single-image treatments, we wanted a robust view of the effects of political microtargeting, so we had several conditions. We paid a few thousand people to take our survey online. Each of these people first answered a bevy of questions about their demographics and ideology, then was subjected to a treatment (more on that in a second), then we asked them a bunch more questions about their ideology. Some of the pre-treatment and post-treatment questions were the same. Using these questions, we could see how much a person's opinion changed because of the treatment.
Treatment Conditions
People can be subject to political messages in a variety of ways. The most common is by reading or hearing one message. To test this, we had a few one-shot conditions:
- One where the respondent read a generic control message about board games.
- One where the respondent read a persuasive political message that was not microtargeted.
- One where the respondent read a persuasive political message that was microtargeted.
Respondents who received a political message read about one of two topics: whether the U.S. should increase/decrease legal immigration, and whether the government should do more/less to prevent k12 teachers from bringing their personal views into the classroom. We chose these topics based on partisan data from Pew. American conservatives tend to have stronger moral feelings about teachers bringing their personal beliefs into the classroom, and American liberals tend to have stronger moral feelings about immigration. All respondents were persuaded counter-attitudinally. That is to say, if the respondent initially favored increasing immigration, they were persuaded to decrease immigration. We persuade counter-attitudinally because that's the direction people can change their mind.
These treatment conditions were interesting, but we were really interested in real-time conversations. This is a new avenue of technology that really couldn't be done just a few years ago. To test this, we had three interactive conditions:
- One where the respondent conversed with an LLM about board games.
- One where an LLM that had the respondent's data tried to persuade them on a political topic in a persuasive conversation.
- One where an LLM that had the respondent's data tried to persuade them on a political topic in using a technique called motivational interviewing.
Motivational interviewing is a technique where the practitioner (here an AI model) converses with someone and tries to get that person to reflect on why they hold certain beliefs. This contrasted with our directly persuasive conversation condition. All of the interactive conditions used the same topics as the one-shot ones.
Results
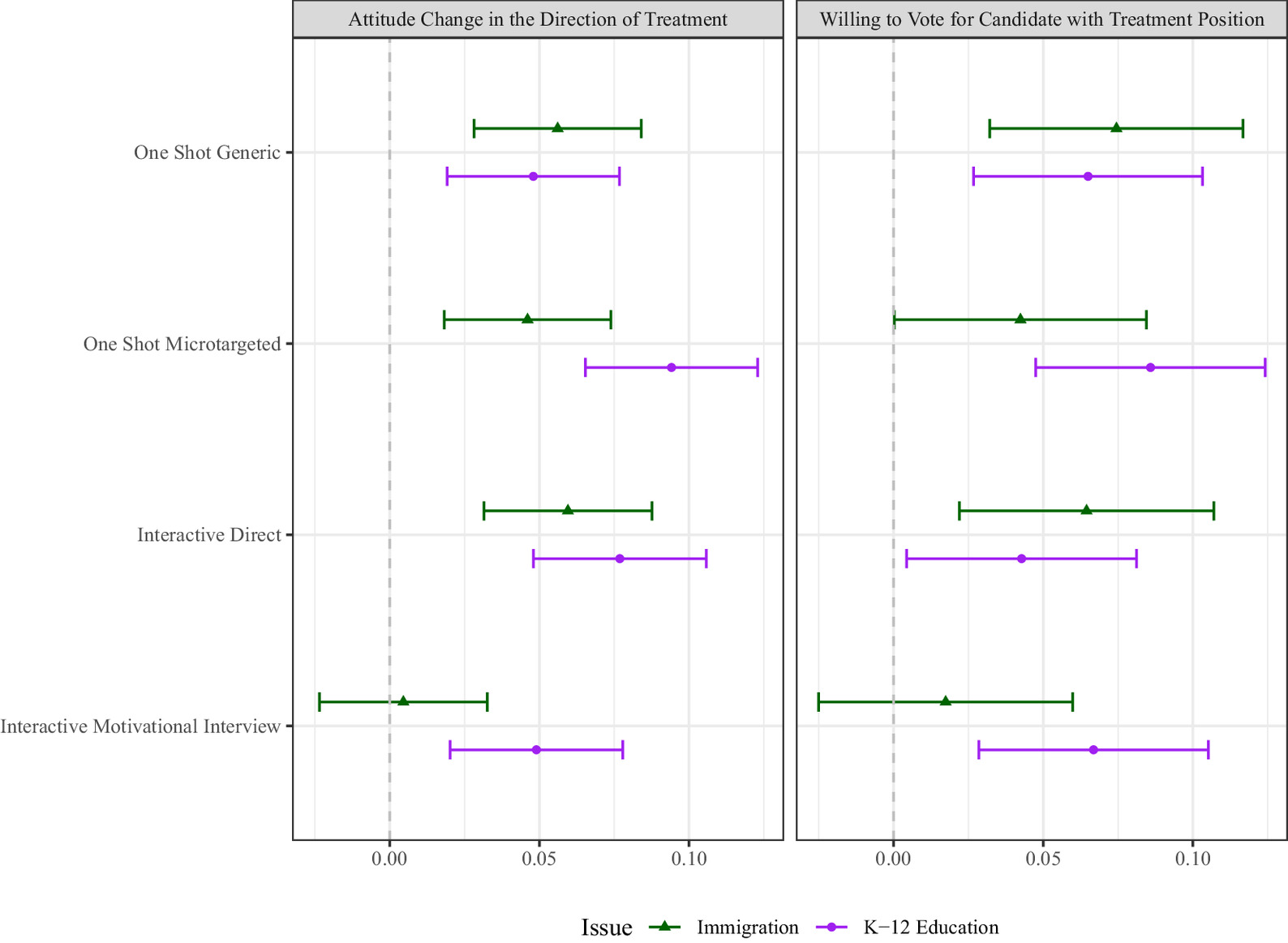
As we can see from the left side of the above figure, our generic and microtargeted one-shot interventions moved people in the direction of treatment. While sometimes a microtargeted message was more persuasive, this difference was not statistically significant. The interactive persuasion was also effective in moving the respondents in the direction of treatment. However, motivational interviewing was less effective in our experiments. Perhaps this is because motivational interviewing causes people to reflect on their current beliefs, without pushing strongly in a direction.
The right side of the figure measures a slightly different question, "How likely would you be to vote for a candidate that says X?" where X is the treatment variable. We asked this to see if moving people's opinion on a topic would translate to changing their voting patterns. Overall, it seems like it would, since both tables match pretty well.
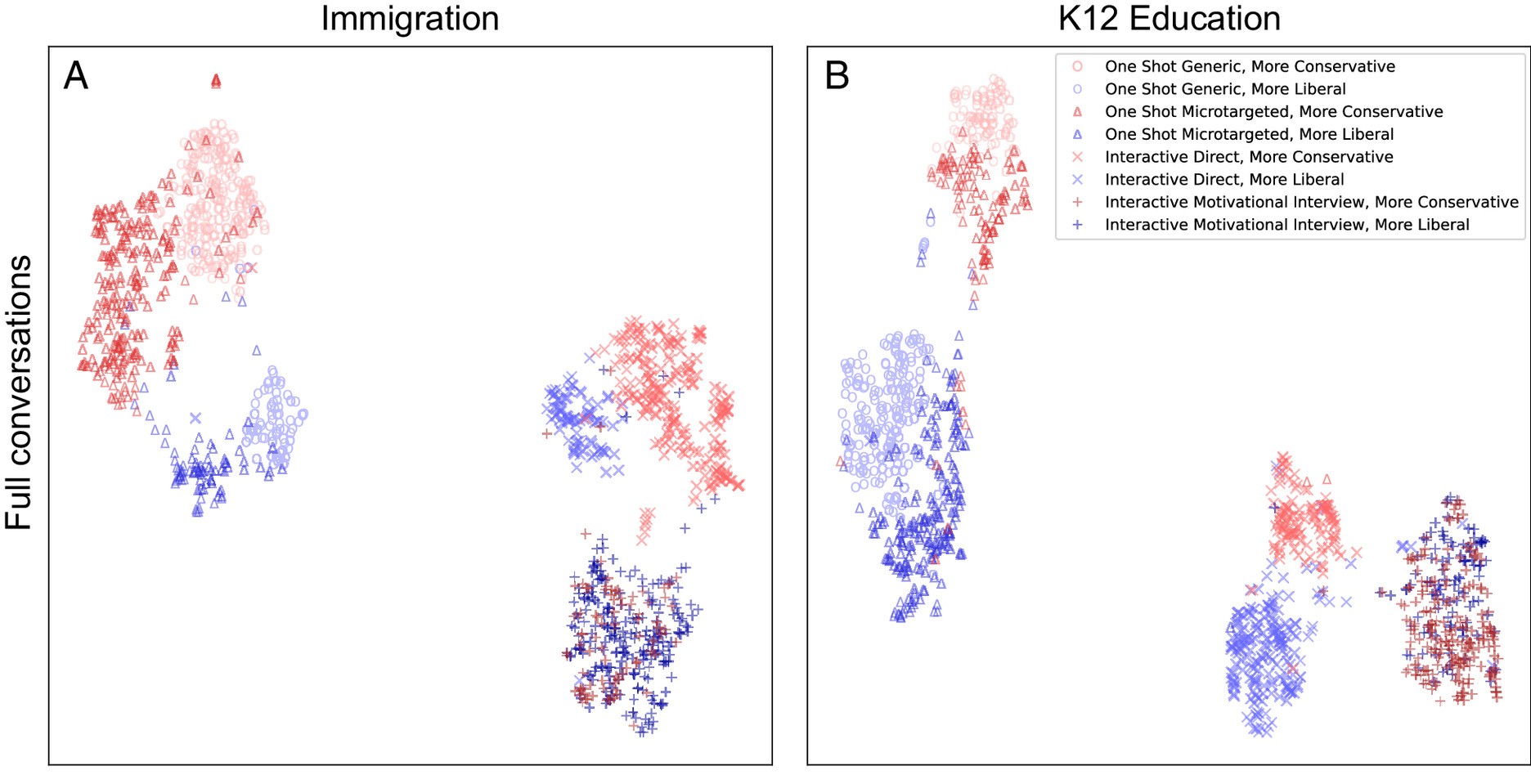
Another question we wanted to answer is, "Are the generated messages saying what we want them to say?" One convenient way to visualize this is by embedding every message generated by the AI in semantic space. Using this technique, similar text is grouped near other, similar texts. In the above figure, each point represents the AI's portion of one treatment conversation. We can see big differences between the one-shot and interactive conditions. We also see clear differences between the conservative and liberal persuasive messages, and less clear but still visible breaks between generic and microtargeted messages. Interestingly, the motivational interviews in both conservative and liberal directions take up roughly the same space. This may be why we saw the smallest effect from this treatment condition.
I made this figure for the paper, so I'm extra proud of it.
Another interesting finding from our study is that while we were able to move people on both of these issues, this did not make them more sympathetic to people with those views. We asked respondents questions about how they felt about people with the opposite view from them. Even when their positon moved towards the opposite, they did not report being more willing to engage with that hypothetical person. While there is an idea that moving people towards the center will help them get along, our data suggest that merely changing people's policy opinions may not change how they relate to others in a positive manner.
Final Takeaways
So what did we learn? LLMs can effectively persuade people on multiple political topics. At least in our treatment, microtargeting doesn't seem to be that much better than generic persuasion. I'm currently working on some follow-up work where I analyze the AI-generated messages and quantify how, exactly, the LLM is microtargeting.
I also learned how long it can take to get something published. We submitted this in time for it to come out ahead of the 2024 election, but it was stuck in review forever. If you want to read the article, you can find it here. We paid to make it open-access, so take advantage!